Generative AI
The Power of Large Language Models in Cognition as a Service
Generative AI is one of the most transformative components of Cognition as a Service (CaaS), enabling machines to create new content, ideas, and solutions autonomously. By leveraging advanced machine learning models, Generative AI drives innovation across industries, empowering organizations to automate creativity, streamline development processes, and enhance decision-making.
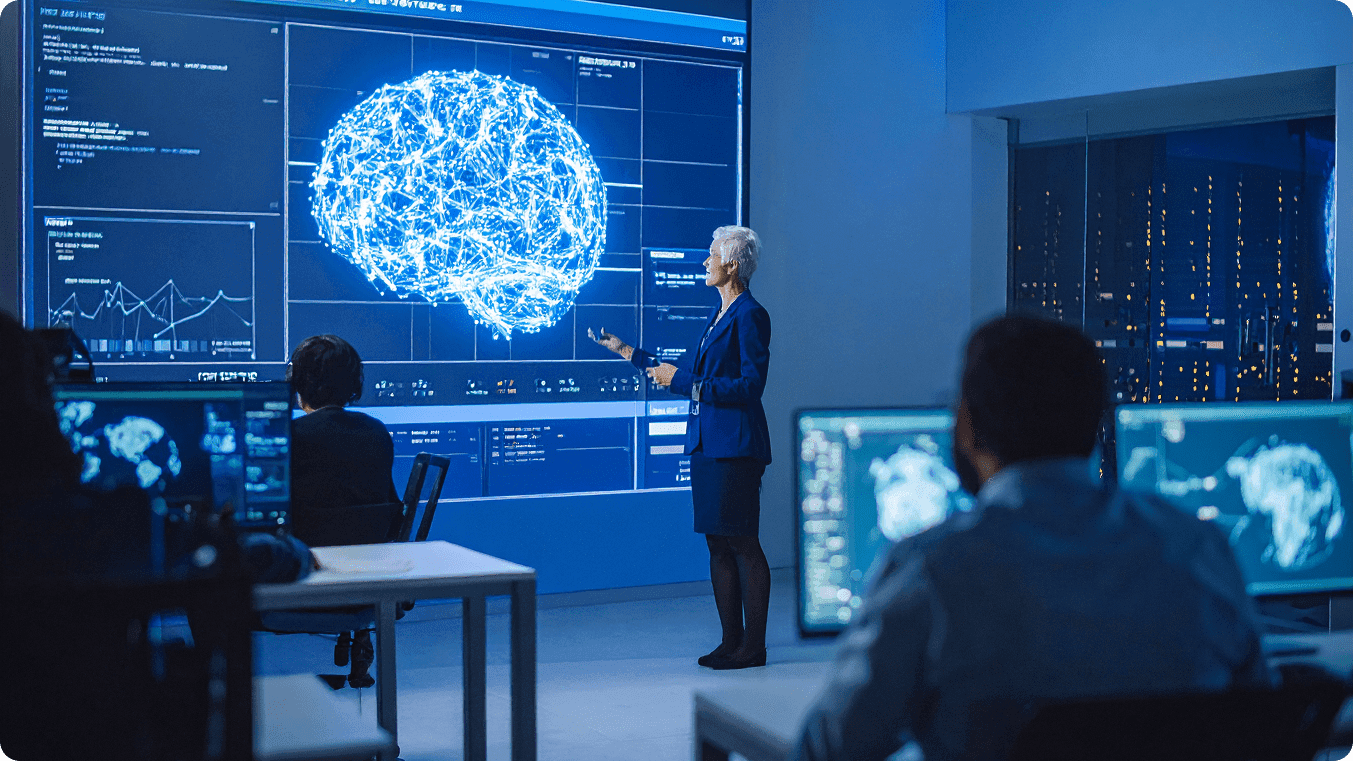
What is Generative AI?
Generative AI refers to a class of artificial intelligence systems that can produce original content, including text, images, audio, code, and even synthetic data. These systems learn patterns and structures from vast datasets and use that knowledge to generate new, contextually relevant outputs.
Within the CaaS framework, Generative AI operates as an on-demand service, allowing organizations to access creative cognitive capabilities without the need to develop or maintain complex AI models in-house.
Key Capabilities of Generative AI
Generative AI models are trained to perform a variety of tasks, such as:
Text
Generation
Automated content creation, report writing, and email drafting
Image and Video Synthesis
Producing realistic visuals for media, design, and simulation
Code Generation
Assisting developers with programming and bug fixing
Synthetic Data Creation
Generating data for model training without compromising privacy
Product Design
Creating innovative product prototypes and simulations
Market Growth and Industry Data
The global market for Generative AI is experiencing rapid expansion. According to Bloomberg Intelligence, the market size is projected to reach $110 billion by 2030, growing at a CAGR of 42%. Adoption rates are particularly high in sectors such as healthcare, entertainment, finance, and manufacturing.
Use Cases and Practical Applications
Healthcare
Pharmaceutical companies use generative AI to accelerate drug discovery. One major firm reduced new drug formulation time by 68% through AI-driven molecular simulation.
Enhances molecular modeling for complex compound identification
Speeds up clinical trial predictions and success rates
Media & Entertainment
Financial Services
Manufacturing
Challenges and Ethical Considerations
While Generative AI offers significant benefits, it also introduces challenges:

Data Privacy & Ownership: Clear policies are needed to address content ownership and ethical use.

Bias & Fairness: Generated content may reflect biases present in training data.

Security Risks: Synthetic data and content can be misused for misinformation or fraud.
Organizations must adopt responsible AI governance frameworks when implementing Generative AI in CaaS environments.
FAQ
What are the benefits of Generative AI in CaaS?
It enables scalable, automated content creation and problem-solving without requiring dedicated AI development teams.
Is Generative AI suitable for regulated industries?
How can organizations manage the risks of Generative AI?
What infrastructure is required to support Generative AI?
Related Insights
Get in touch